From data to insights: Leveraging outlier analysis for financial forecasting
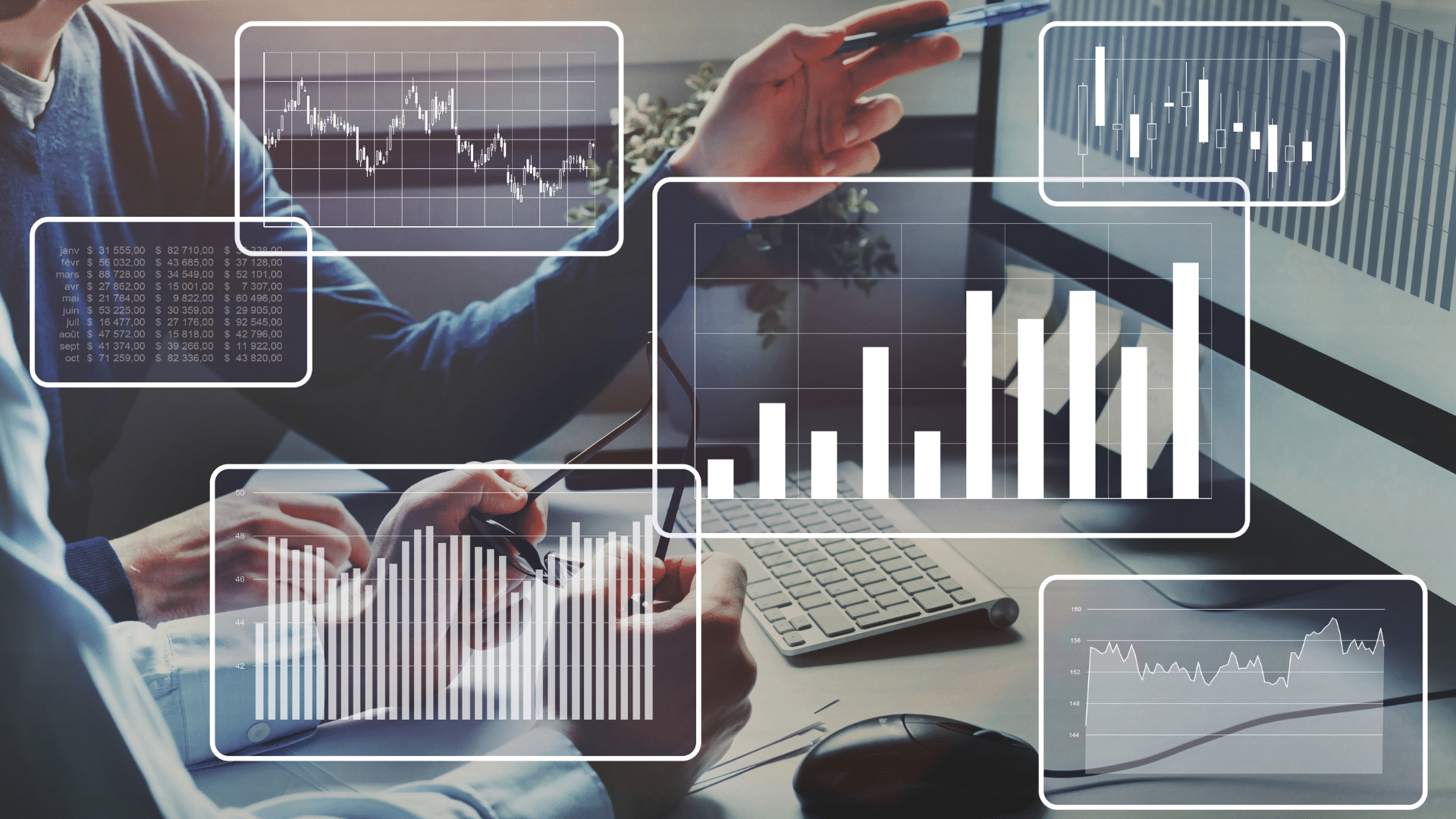
The value of unusual developments for predictive forecasting, financial planning and economic decision-making
What if you could decipher the underlying causes and effects of unusual shifts in your financial KPIs?
In today’s information-driven world, where vast amounts of data, powerful algorithms and flexible reports are at the fingertips of financial leaders, it’s often the anomalies and unexpected shifts that provide the most information for effective decision-making.
The urgent requirement for precision in outlier identification, analysis and management cannot be understated. These anomalies, often elusive in nature, can either be windows to untapped opportunities or hidden threats. Delving deep into the realm of outlier analysis, especially within financial data, can profoundly enhance forecasting and facilitate well-informed and data-driven decision-making.
Many financial departments struggle with escalating demands, often handling heightened responsibilities with limited resources. Over the last few years, their challenges have been intensified by a volatile economic environment and growing regulatory requirements.
With the dawn of cutting-edge analytics, distilling valuable insights from large datasets has become remarkably fast and effective. So, the question arises, how can financial leaders tap into outlier detection to transform potential pitfalls into actionable intelligence?
By synergizing advanced analytics and business experience, companies can leverage profound insights with unmatched effectiveness. By using real-time analytics and outlier detection algorithms, a sudden surge in operational costs can be identified and analyzed to investigate its causes and deploy effective counter measures. The possible crisis can be managed proactively to minimize its impact. Adopting such forward-thinking strategies can supercharge decision-making processes, potentially providing an unmatched competitive advantage.
Outlier detection, in essence, serves as a sentinel, enabling businesses to act before minor problems evolve into major crises. For financial professionals, outlier detection might also unveil unexpected shifts in revenue, expenses or cash flows. Such deviations could flag errors in financial ledgers or hint at malfeasance like unwarranted expenditures. Outliers could also be the effect of seasonal fluctuations during festive seasons, an abrupt sales boom triggered by a groundbreaking product launch, macroeconomic shifts, data recording errors, or even covert competitor maneuvers like pricing recalibrations or marketing campaigns – nuances that often elude human detection. By pinpointing these outliers and understanding their drivers and impacts, analytics-driven professionals can estimate their effects and improve the forecast quality to support decision-making and guide future strategies.
Understanding outliers in financial time series data
An observation that deviates so much from other observations as to arouse suspicions that it was generated by a different mechanism.
Outliers are data points that differ significantly from the rest of the dataset. They may indicate a measurement or data entry error, or they may be a legitimate but unusual data point. Effectively identifying and managing outliers is essential for the accuracy and reliability of financial analysis, playing a key role in data cleaning and preparation.
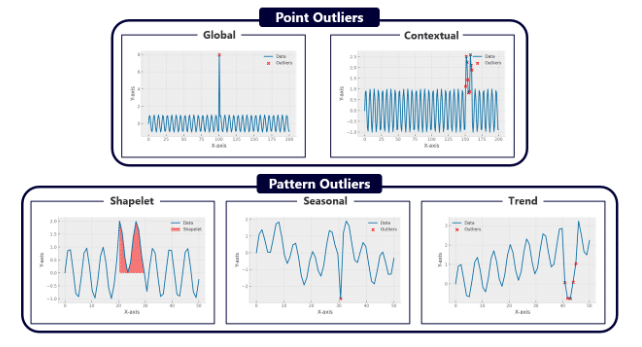
Figure 1: Different types of outliers in time series datasets
In the realm of time series data, outliers manifest in two primary forms: pointwise and pattern-wise anomalies.
Pointwise anomalies: These singular data points are distinguished either on a global scale (in comparison to the entire dataset) or within their immediate context (relative to adjacent points). For example, a sudden and atypical surge in a company’s operational expenses on a particular day would typify a contextual anomaly.
Pattern-wise anomalies: These anomalies exhibit distinctive patterns, potentially appearing as uncommon shapelets, irregular seasonal behaviors, or unexpected trend trajectories. An instance of this could be a steady, weekly escalation in sales figures that strays from the expected seasonal norm.
The benefits of outlier detection in financial data
In the corporate arena, outlier detection plays a dual role as both a protective measure and a tactical instrument.
Risk management: Outlier detection acts as a preemptive alert system, spotlighting potential hazards, such as atypical transaction patterns that could signify fraudulent activities.
Revenue enhancement: Outliers aren’t always a cause for concern. Sometimes, a sharp rise in product demand may reveal an overlooked market opportunity, thereby informing decisions on resource distribution.
Operational streamlining: Outlier detection is instrumental in pinpointing inefficiencies. For example, the detection of a drop in productivity in a department can lead to targeted improvements, potentially necessitating adjustments in staffing, training, or operational procedures.
Strategic reorientation: Persistent deviations in data from forecasted strategic outcomes can be an indicator to reevaluate and possibly modify business strategies, particularly in dynamic market environments.
Implementing outlier detection in financial processes serves a dual purpose: It protects against unforeseen risks and enhances opportunities for positive outcomes.
Outlier detection: The four steps to data-driven impact
The ever-changing landscape of financial data necessitates that outlier analysis be an ongoing endeavor, involving the use of unsupervised models to recognize emerging patterns. When dealing with time series outliers, there are two primary categories, each dependent on the analyst’s focus or the specific context.
Firstly, observations that constitute noise, errors, or irrelevant data, and hold no interest for the analyst, should be removed or corrected to enhance data quality. For instance, rectifying errors is crucial for more accurate forecasting, as the primary goal here is to enhance predictive accuracy.
Secondly, if the outlier is significant and warrants attention, the approach unfolds in several steps:
- Identification: Ascertain whether it’s genuinely an outlier or merely a data anomaly.
- Causal analysis: Delve into the reasons behind its occurrence.
- Business impact assessment: Evaluate how it influences key metrics like sales or profitability.
- Actionable response: This may involve implementing control measures or revising financial strategies.
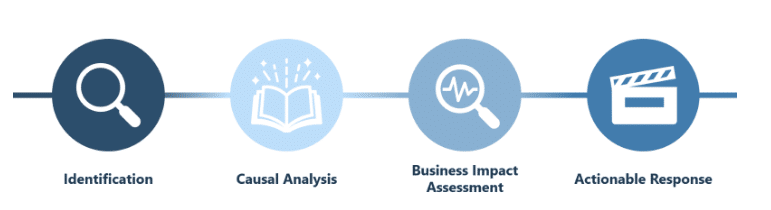
Figure 2: The four steps to data-driven impact
In summary, mastering outlier analysis transcends a mere technical pursuit; it emerges as a strategic instrument for today’s financial leaders. By adeptly navigating the intricacies of outlier detection, organizations can convert seemingly random data points into valuable insights. This not only shields against unexpected financial risks but also reveals latent opportunities, fostering informed, data-driven decision-making. In a world increasingly driven by data, the capacity to effectively interpret and leverage these outliers is a definitive factor in securing a competitive advantage. Outlier analysis thus evolves from a mere analytical tool to a beacon of financial foresight and precision.
Looking to transform unforeseen data fluctuations into strategic assets? Explore the full potential of your financial forecasting with AIVIAN`s advanced outlier detection capabilities. Reach out to us for a complimentary consultation and begin your journey towards data-driven excellence.
Tim Fritzsche has been working as a consultant at verovis since 2022, covering all topics related to AI & Machine Learning. His focus is on automating processes and enriching them with „cutting edge“ technologies to provide his clients with the relevant informational advantage.
Questions for our experts? Get in touch with us!
Von Team #valantic Digital Finance